Among other industries, it is especially surprising to see AI transforming the healthcare sector at a rapid pace. AI in healthcare management is driving revolutions in diagnostics, patient management, and predictive analytics. Unlike pre-AI times, AI-powered applications now have the bandwidth to deal with large datasets, complex algorithms, and real-time insights which in turn simplifies medical decision-making. Let's see the different ways AI is leading this transformation with some real-time instances from the Techjays’ foray into AI in healthcare projects.
1. Diagnostic Applications: Unleashing the Power of Machine Learning
The current role of AI in enhancing medical diagnostics almost entirely lies with machine learning (ML) models - and in it, Convolutional Neural Networks (CNNs).

CNN has shown ground-breaking results in analyzing medical images like radiology reports. AI models that are trained on humongous amounts of radiology reports can detect pathologies . Be it tumors or fractures, these models are trained to read reports with accuracy rates on par with, or even exceeding, those of human radiologists.
Let's see some more specific examples.
Computer Vision in Medical Imaging: Similar to radiology reports, AI models can also process CT scans, MRIs, and X-rays using advanced feature extraction techniques. Data augmentation is also often employed to improve the analysis of limited medical datasets.
Natural Language Processing (NLP) for Electronic Health Records (EHRs): In the process of analyzing medical records, unstructured texts in electronic health records may be a big hindrance. NLP algorithms can extract clinical information from such unstructured text in EHRs - thereby enlightening physicians with important diagnostic insights. Today, transformer models like BERT and GPT are being fine-tuned to understand medical terminologies. This can help analyze medical records - unstructured texts in electronic health records.

Genomic Data Analysis: AI is revolutionizing genomics as well, which is the study of an organism’s genetic material and how that information can be applied in cases like identifying disease predispositions. Analyzing genetic sequences is crucial in finding genetic mutations resulting in hereditary diseases and algorithms like Random Forests. Deep learning networks are showing exceptional efficiency in recognizing and analyzing them. With the power of AI, we are very close to looking at a future free of genetic diseases.
Potential Challenges:
Data Variability: The multiplicity of medical imaging devices and variations in data labeling is currently a challenge for using general AI models. Domain adaptation techniques may be required in such cases to handle variability.
Regulatory Constraints: AI systems need to undergo rigorous validation to meet the FDA's guidelines. This necessitates stringent performance monitoring to ensure safety and effectiveness.
Next, let's look at how AI transforms Patient Management processes
2. Patient Management: Elevating Personalized and Predictive Care
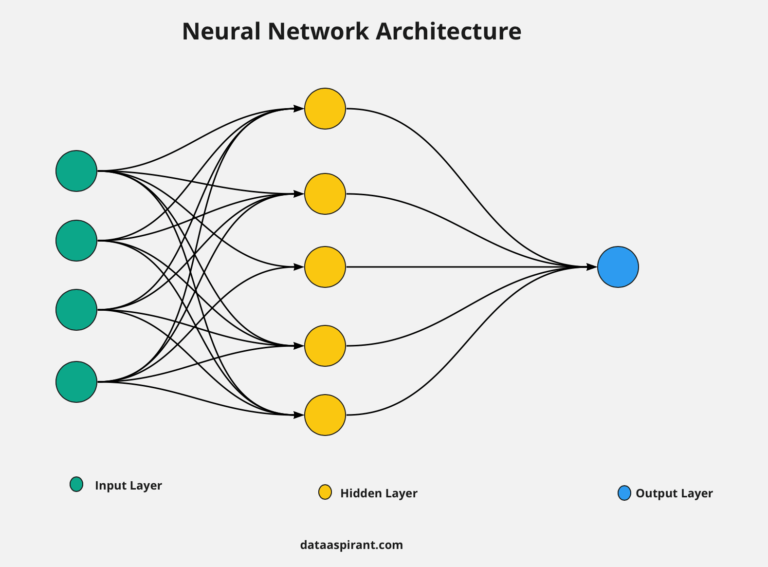
Predictive modeling is a significant benefit of AI in healthcare where AI systems can forecast disease progression and even suggest personalized treatment courses. Along with these two, Recurrent Neural Networks (RNNs) and Long Short-Term Memory (LSTM) models can analyze data from wearable monitoring devices that track chronic conditions and send alerts based on prescribed levels.
Predictive Analytics: AI algorithms in healthcare projects can analyze patient history and predict patient outcomes. Today, deep reinforcement learning and Gradient boosting machines are used widely in conditions like sepsis or heart failure in making real-time decisions.
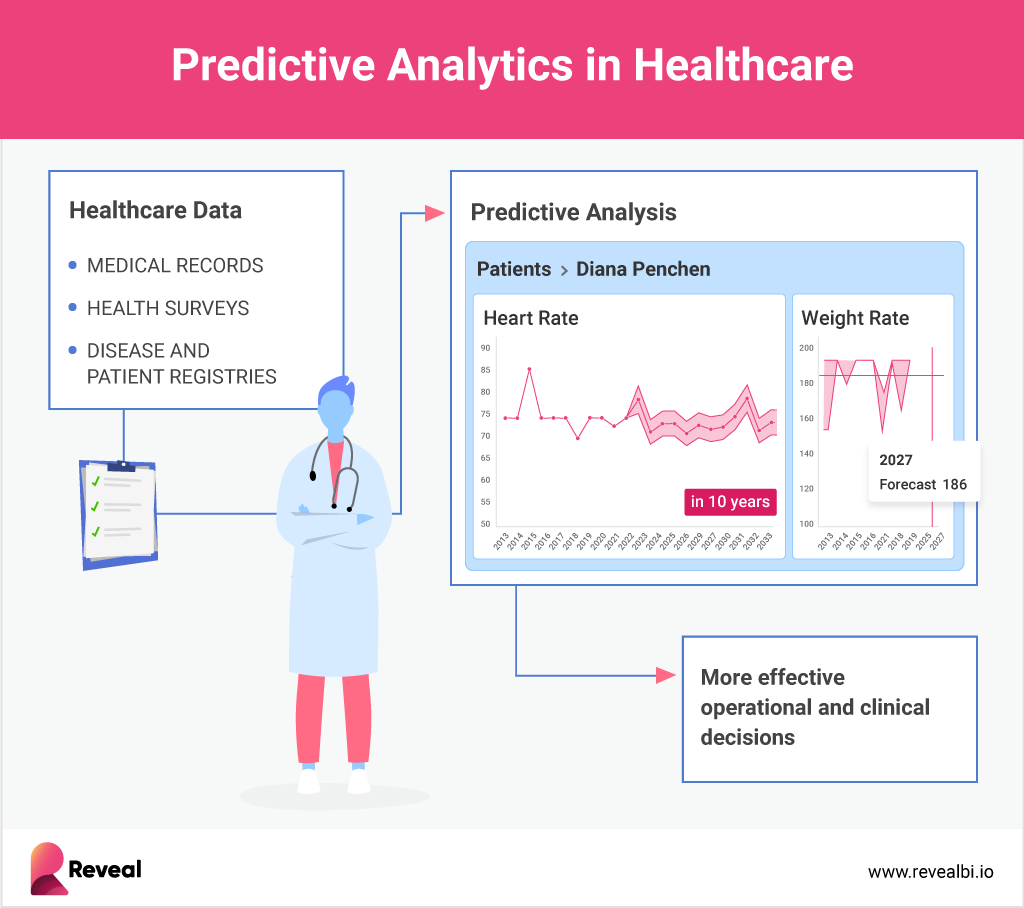
- Virtual Health Assistants: Chatbots in AI health apps support patients by answering medical queries and assisting in scheduling appointments. These models can be and are usually trained on extensive volumes of medical literature so that it is adept in understanding and responding in a clinical context.
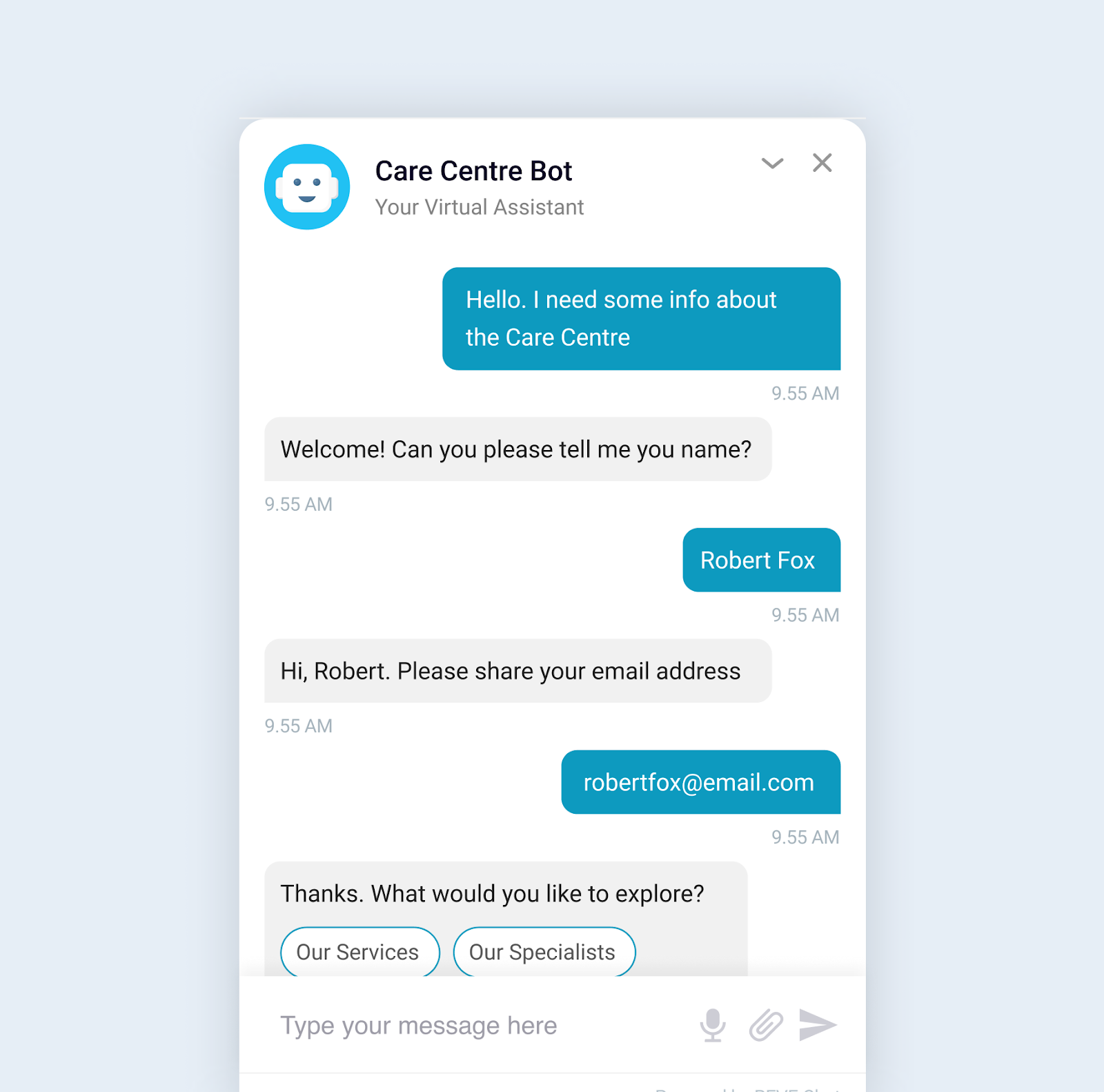
- Telemedicine Platforms: AI is an expert in automating administrative tasks such as transcribing consultations and triaging patients. Transcribing consultation is based on using speech-to-text algorithms. Triaging patients works by using Bayesian networks on their symptoms.
Challenges in Patient Management:
- Data Privacy and Security: Medical records are highly sensitive data and hence handling them requires complying with stringent regulations like HIPAA. Such regulations are inevitable while incorporating AI in healthcare projects and require implementing robust encryption methods to preserve patient confidentiality.
- Bias and Fairness: Training AI models need to be a very careful exercise. Training on imbalanced datasets may perpetuate healthcare disparities. To mitigate such biases it is important to employ techniques like model fairness optimization and adversarial debiasing.
Model Fairness Optimization is a set of techniques aimed at reducing biases in machine learning models to ensure fair and equitable treatment across varying groups.
Adversarial Debiasing is also an in-processing technique used to reduce model bias where a secondary model is trained simultaneously to predict the characteristic that should not unfairly influence predictions.
3. AI in Clinical Workflows
Interoperability with existing healthcare systems is an inevitable mantra when it comes to integrating AI into clinical environments.

Fast Healthcare Interoperability Resources (FHIR) standards facilitate data exchange between AI and EHR systems. However, in a practical scenario, real-time data processing can show latency. This can be addressed using high-throughput, low-latency data architectures.
Edge Computing in Healthcare: Edge computing is essential in healthcare to manage the volume and velocity of incoming data. In edge computing, data is processed closer to its source, thus reducing latency and enhancing response times. This is especially important for critical applications like patient monitoring in intensive care units.

- Federated Learning: This is a decentralized ML approach that trains AI models across multiple hospitals without compromising sensitive data. Here, to ensure data privacy while training models, techniques like differential privacy and secure multiparty computation are used.
Challenges in Clinical Integration:
- Interoperability: AI in healthcare projects need to adhere to HL7 standards for data exchange and must be compatible with legacy systems. For this, middleware solutions and API-driven integrations might be necessary.
- Model Interpretability: Clinicians and practitioners need to understand the logic behind AI-driven recommendations and for this, might often need explainable AI (XAI) models. SHAP (SHapley Additive exPlanations) and LIME (Local Interpretable Model-Agnostic Explanations) are techniques that can provide transparency in making model decisions.
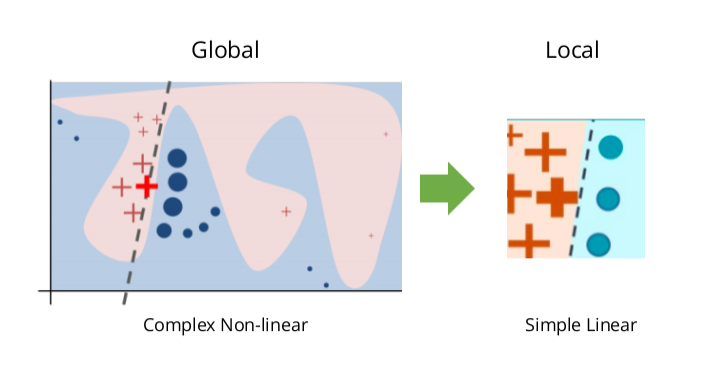
Courtesy: c3.ai
4. Ethical Considerations and the Future
As we deal with incorporating AI in healthcare projects, it is crucial to remember that it is a sensitive domain and that there are significant ethical questions about accountability and transparency. It is essential to establish ethical frameworks and guidelines for the responsible deployment of AI technologies.
- Explainable and Transparent AI: Developing interpretable models is more of an ethical imperative as much as it is a technical requirement. This can ensure trust in AI systems. To create transparent deep-learning models, research into XAI (explainable AI) is underway.
- Autonomous Decision-Making: Giving AI models the imperative to make autonomous decisions always raises liability concerns. Ensuring human oversight and developing ethical guidelines for AI deployment in clinical settings become critical.
Healthcare Tech at Techjays:
- PepCare - Streamlining Patient-Doctor Communication
Our healthcare tech client, Pepcare, required a platform to enhance collaboration between doctors and patients and dons multiple collaboration and referral management tools by leveraging wisely on technology and AI.
The vision of the creators was to create a seamless solution that simplifies administrative tasks, facilitates virtual consultations, and expands the network of dental practitioners.
The platform required to digitize every communication mode and medical document and transform the patient referral process. This was to help doctors to refer patients to other doctors and share all medical records and patient history through the HIPAA-secure platform.

Techjays got into creating the platform, where apart from providing seamless working, it was also imperative to address the increasing number of cyber threats and ensure security and privacy as the platform dealt with extremely sensitive medical data.
Additionally, the need to include additional features such as HIPAA compliance required us to go the extra mile.
Our platform enabled Medical practitioners to create groups among themselves where they can discuss cases, collaborate, and utilize expertise in different specialties.
In addition, the platform also included a feature called the Patient Vault for patients, where patients can have all their old and new medical records, prescriptions, and reports, minimizing any chance of misplacing but accessible whenever needed. This turned out to be a great way to maintain a patient's medical history.
The platform also includes all features necessary for doctors to impart virtual care, especially for follow-up evaluations - in-built screen recording to explain medical records like X-rays and upload educational and best practice videos so that patients can access them at any time.
Today, using our platform both patients and doctors can create accounts, and patients can search for doctors and look up ratings and reviews too.
Next up, it is an AI-powered level-up for PepCare built by Techjays!!!
- Aidendo - Bolstering Endocrinology
In this journey, the Advanced Institute for Diabetes and Endocrinology required Techjays to create a platform specifically for endocrinology patients, a platform that would capture patients’ health metrics, such as blood pressure, weight, blood sugar, etc.
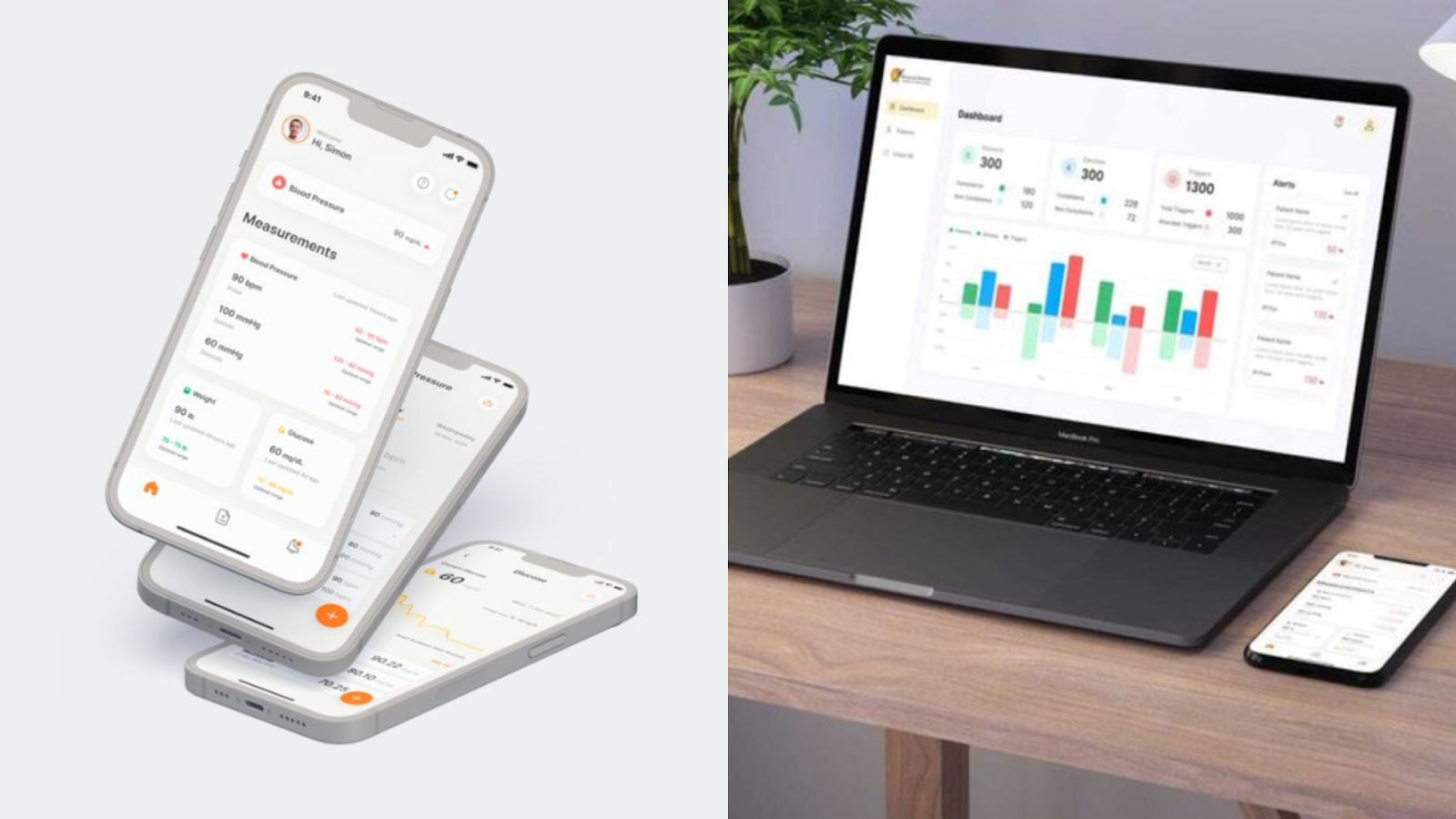
The experts at Techjays created an interface where output from a physical monitoring device was synced to a patient’s smartphone, and from there to a dashboard was created for the endocrinologists.
The product does end-to-end patient handholding from prescriptions to sending alerts when the metrics go beyond stipulated levels. This product is also gearing up to incorporate AI-powered processes now.
The Inevitable Foray into AI
The unprecedented potential of AI in healthcare management be it in diagnostics, patient management, and personalized medicine is amazing. However, many technical challenges, particularly those ensuring data security and upholding ethical standards will require addressing. As research and technology evolve, collaboration between AI developers, healthcare professionals, and regulators will be essential to realize the full potential of AI in healthcare.